Guest Blog by Jeffrey L Mckinstry
We presented our latest work on low precision inference at the Energy Efficient Machine Learning and Cognitive Computing Workshop at NeurIPS 2019 in Vancouver. We show that 4 bits suffice for classification for a wide range of Deep Networks.
Title: Discovering Low-Precision Networks Close to Full-Precision Networks for Efficient Inference
Authors: Jeffrey L. McKinstry, Steven K. Esser, Rathinakumar Appuswamy, Deepika Bablani, John V. Arthur, Izzet B. Yildiz, Dharmendra S. Modha
Abstract: To realize the promise of ubiquitous embedded deep network inference, it is essential to seek limits of energy and area efficiency. Low-precision networks offer promise as energy and area scale down quadratically with precision. We demonstrate 8- and 4-bit networks that meet or exceed the accuracy of their full- precision versions on the ImageNet classification benchmark. We hypothesize that gradient noise due to quantization during training increases with reduced precision, and seek ways to overcome this. The number of iterations required by SGD to achieve a given training error is related to the square of (a) the distance of the initial solution from the final and (b) the maximum variance of the gradient estimates. Accordingly, we reduce solution distance by starting with pretrained fp32 baseline networks, and combat noise introduced by quantizing weights and activations during training by training longer and reducing learning rates. Sensitivity analysis indicates that these techniques, coupled with activation function range calibration, are sufficient to discover low-precision networks close to fp32 precision baseline networks. Our results provide evidence that 4-bits suffice for classification.
Link: https://www.emc2-workshop.com/assets/docs/neurips-19/emc2-neurips19-paper-11.pdf
To harness the power of deep convolutional networks in embedded and large-scale application domains, for example in self-driving cars, requires low-cost, energy-efficient hardware implementation. One way to reduce energy and hardware cost is to reduce the memory usage of the network by replacing 32-bit floating point weights and activations with lower-precision, such as 8- or even 4-bits. If such low-precision networks were just as accurate as the 32-bit floating point (fp32) version, the energy and system cost savings would come for free. Unfortunately, the accuracies are lower for the 8-bit networks than for the corresponding full-precision net (see Table 1, from the workshop paper). The situation is even worse for 4-bit precision. For the ImageNet classification benchmark, no method has been able to match the accuracy of the corresponding full-precision network when quantizing both the weights and activations at the 4-bit level. Closing this performance gap has been an important open problem until recently, when we showed that our Fine-tuning After Quantization (FAQ) technique could match the full-precision networks for ResNet-18, -34, and -50 at 4-bit precision. At the conference we presented an update showing that 4-bits suffice for a wide range of deep networks (Table I).
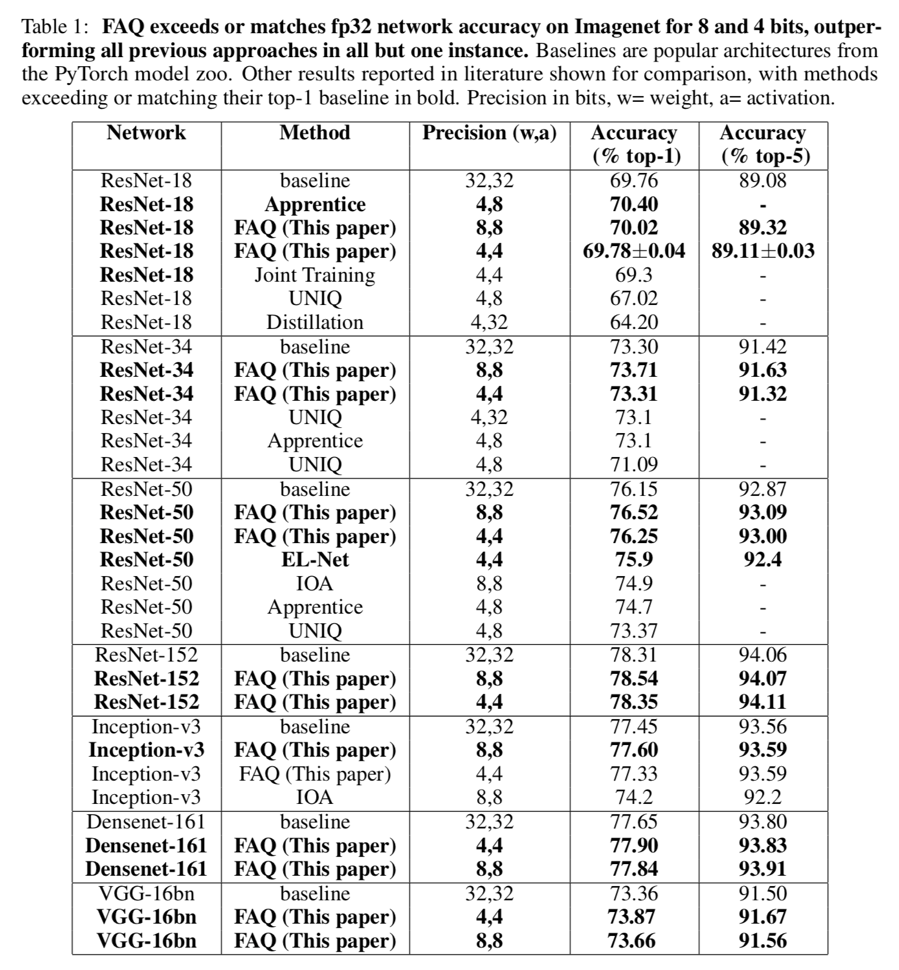
Guided by theoretical convergence bounds for stochastic gradient descent (SGD), we propose fine-tuning, training pretrained high-precision networks for low-precision inference, by combating noise during the training process as a method for discovering both 4-bit and 8-bit integer networks. We evaluate the proposed solution on the ImageNet benchmark on a representative set of state-of-the-art networks at 8-bit and 4-bit quantization levels (Table 1). Contributions include the following.
- We demonstrate 8-bit scores on ResNet-18, 34, 50, and 152, Inception-v3, Densenet-161, and VGG-16 exceeding the full-precision scores after just one epoch of fine-tuning.
- We present evidence that 4 bits suffice for classification; fully integer deep networks match the accuracy of the original full-precision networks on the ImageNet benchmark.
- We demonstrate that reducing noise in the training process through the use of larger batches provides further accuracy improvements.
- We find direct empirical support that, as with 8-bit quantization, near optimal 4-bit quantized solutions exist close to high-precision solutions, making training from scratch unnecessary.
Fine-tuning after Quantization (FAQ)
Our goal has been to quantize existing networks to 8 and 4 bits for both weights and activations while achieving accuracies that match or exceed the corresponding full-precision networks. For precision below 8 bits, the typical method that we used in our prior work (Esser et al, 2016) is to train the model using SGD while rounding the weights and neuron responses.
It has been known for some time that 8-bit networks are able to come close to the same accuracy as the corresponding 32-bit network, even without retraining, indicating that 8-bit networks have approximately the same capacity as the fp32 networks. Very recently it was shown, for ResNet-18 and 50, that networks with 4-bit weights and activations could come within 1% of the accuracy of the fp32 networks when training from scratch, suggesting that 4-bit networks may also have the same capacity as the corresponding fp32 networks. We therefore looked for a way to train low-precision networks to match or even exceed the corresponding high precision networks. Our working hypothesis is that noise introduced by quantizing the weights and activations during training is the limiting factor, and we looked for ways to overcome it.
Some hints come from a theoretical analysis regarding how long it takes to train a network using SGD. The number of iterations required increases with the square of (a) the distance of the initial solution from the final plus (b) the maximum variance of the gradient estimates. This suggests two ways to minimize the final error. First, start closer to the solution. We therefore start with pretrained models available from the PyTorch model zoo (https://pytorch.org/docs/stable/torchvision/models.html) for quantization, rather than training from scratch. Second, minimize the variance of gradient noise. To do this, we combine techniques to combat noise: learning rate annealing to much lower learning rates with longer training time. In addition, we automatically calibrate the quantization parameters for weights and activations for each layer given the data distributions and the precisions. We refer to this technique as Fine-tuning after quantization, or FAQ. Table 1 shows that the proposed method outperforms all other algorithms for quantization at 8- and 4-bits and can match or exceed the accuracy of the corresponding full-precision networks in all but 1 case.
We found further empirical support that starting from a pretrained network was indeed helpful. The full-precision network weights were very similar to the final weights after running FAQ on ResNet-18. Given that a good 4-bit network was found close to the full-precision network suggests that it is unnecessary, and perhaps wasteful to train from scratch.
FAQ is a principled approach to quantization. Given these results on state-of-the-art deep networks, we expect that it will generate much interest, and replace existing methods. Our work here demonstrates 8-bit and 4-bit quantized networks performing at the level of their high-precision counterparts can be created with a modest investment of training time, a critical step towards harnessing the energy-efficiency of low-precision hardware.