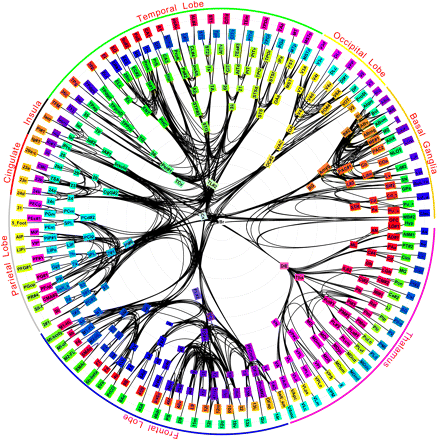
Today, July 27, Proceedings of the National Academy of Sciences of the USA (PNAS) published the paper entitled “Network architecture of the long-distance pathways in the macaque brain” by Raghavendra Singh (IBM Research – India) and myself. The main paper is 6 pages long and the appendix is 57 pages.
Figure 1 of main paper: Visualized below is the long-distance network of the Macaque brain spanning the cortex, thalamus, and basal ganglia that captures many aspects of the cumulative contribution of a whole community of neuroanatomists over the last half-century. The network has 383 brain regions and 6,602 long-distance connections. High-resolution version of the figure is here.
We have successfully uncovered and mapped the most comprehensive long-distance network of the Macaque monkey brain, which is essential for understanding the brain’s behavior, complexity, dynamics and computation. We can now gain unprecedented insight into how information travels and is processed across the brain.
The brain network is organized and can be studied at multiple scales, connecting single neurons, small populations of neurons, brain regions, larger brain structures and functional subsystems together. Short-distance gray matter connections constitute “local roads” within a brain region and its sub-structures. We have focused on the long-distance brain connections that travel through the brain’s white matter, which are like the “interstate highways” between far-flung brain regions.
Building on the publicly available database called Collation of Connectivity data on the Macaque brain (CoCoMac), which compiles anatomical tracing data from over 400 scientific reports published over the last half-century, we have studied four times the number of brain regions and have compiled nearly three times the number of connections when compared to the largest previous endeavor. Our data may open up entirely new ways of analyzing, understanding, and, eventually, imitating the network architecture of the brain, which according to Marian C. Diamond and Arnold B. Scheibel is “the most complex mass of protoplasm on earth-perhaps even in our galaxy“.
Key findings:
“Network”:
Our network consists of 383 hierarchically organized regions spanning cortex, thalamus, and basal ganglia; models the presence of 6,602 directed long-distance connections; is nearly three times larger than any previously derived brain network; and contains subnetworks corresponding to classic corticocortical, corticosubcortical, and subcortico-subcortical fiber systems.
“Core sub-network“:
The brain network contains a tightly integrated core that might be at the heart of higher cognition and even consciousness. Strikingly, this discovery aligns remarkably well with three decades of behavioral imaging studies that exhibit a “task-positive” network implicated in goal-directed performance and a “task-negative” network activated when the brain is withdrawn and at wakeful rest.
“Topological Centrality”:
Much as search engines uncover highly ranked web-pages of the world-wide-web using network theory, by ranking brain regions, we found evidence that prefrontal cortex is a topologically-central part of the brain that might act as an integrator and distributor of information.
“Degree distribution”:
The degree distribution is a key signature that provides clues about the possible evolution of the network. The brain network does not appear to be “scale-free” like the web’s social networks, which are logical and can grow without constraints, but seems to be “exponential” like air traffic networks, which are physical and must satisfy resource constraints. This finding will help us design the routing architecture for a network of cognitive computing chips.
What was previously scattered across 410 papers, and limited to neuroanatomists specializing in the wetware of the experimental animal is now unified and accessible to network scientists who can unleash their algorithmic software toolkits. The network opens the door to the application of large-scale network-theoretic analysis that has been so successful in understanding the internet, metabolic networks, protein interaction networks, various social networks, and in searching the world-wide web. The network will be an indispensable foundation for clinical, systems, cognitive, and computational neurosciences as well as cognitive computing.
To paraphrase Lincoln, the data is of the monkey, by the people, and for the people. We have elected to share it with the entire community.
Figure S6 of the appendix: Visualized below is a hierarchical macaque brain map consisting of 383 regions in brain (Br) that is divided into cortex (Cx), diencephalon (DiE), and basal ganglia (BG). Further, cortex is divided into temporal lobe (TL#2), frontal lobe (FL#2), parietal lobe (Pl#6), occipital lobe (OC#2), insula (Insula), and cingulate cortex (CgG#2), and so on. Each brain region is represented via its acronym or abbreviation enclosed in a small colored rectangle. The brain regions in the three outermost circles are leaves that cannot be further subdivided. A color wheel is used for better discrimination amongst brain regions. For the leaf brain regions in the two outermost circles, the color wheel is rotated by 120 degrees and 240 degrees. High-resolution version of the figure is here.
Figure 2 of the main paper: Visualized below is the innermost core of that is a central sub-network that is far more tightly integrated than the overall network, information likely spreads more swiftly within the innermost core than through the overall network, the overall network communicates with itself mainly through the innermost core, and the innermost core contains major components of the task-positive and task-negative networks derived via functional imaging research. High-resolution version of the figure is here. One of our mentors, Professor Kenneth Kreutz Delgado, upon seeing this picture dubbed it “The Mandala of the Mind“.
We were inspired by Poincare who said: “The Scientist … studies nature because he takes pleasure in it; and he takes pleasure in it because it is beautiful. If nature were not beautiful, it would not be worth knowing and life would not be worth living.” In creating the network visualization, we strove to express the truth in artistic terms and to seek beauty in science.
For the technically interested reader, here is a detailed powerpoint slide show with voice narration (60 slides, ~52 minutes, ~50 MB). This may take some time to download given the size.
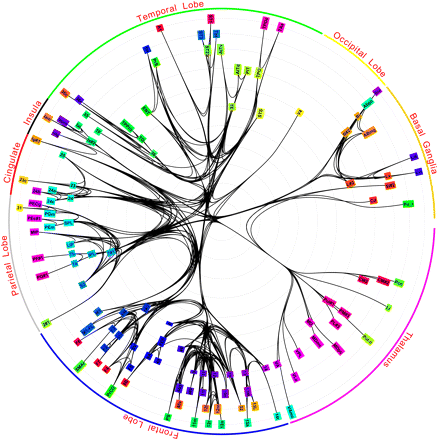